AI Efficiency: The Next Frontier for Enterprise AI Adoption
Written byArvind Ayyala
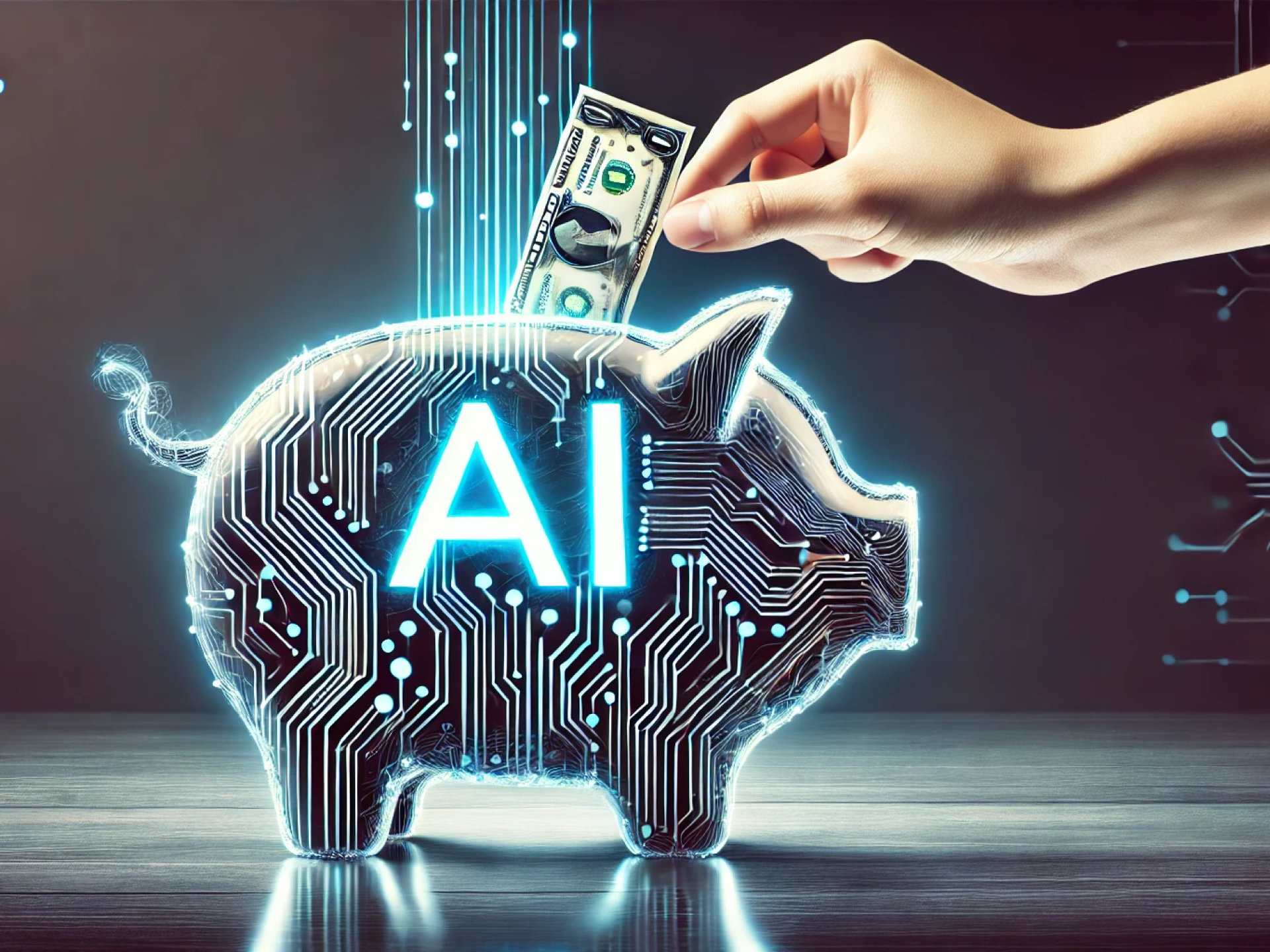
As AI continues to revolutionize industries, enterprises are increasingly looking to harness its power and put it into production for their businesses. However, despite the declining costs of AI models themselves, many organizations still struggle with the overall expense of deploying AI within their infrastructure and struggle to gain the desired efficiencies.
While the availability and capabilities of AI models continue to expand rapidly, the real expense for enterprises often lies not in the models themselves, but in the infrastructure required for training and inference of these models at scale. Training large AI models requires substantial computational resources, talent and time, often translating to hefty cloud computing and manpower bills. Similarly, inference—the process of using trained models to make predictions or decisions—can become a significant ongoing expense, especially for high-volume applications. Custom AI solutions can range from $10,000 to over $500,000, with ongoing operational costs potentially reaching 50-200% of initial development costs.
A. Controlling the Costs
Several innovative companies are pushing the boundaries of AI efficiency, developing technologies and platforms that address the cost and performance challenges faced by enterprises.
1. Deepseek Shines Light on Reinforcement Learning & Synthetic Data
Deepseek’s recent AI reasoning progress gave a jolt to Silicon Valley and technology stocks – on the basis of ~$6M pre-training run costs to create a reasoning model that performs well on varying benchmarks and against OpenAI and Anthropic. The underlying innovation was a combination of chain-of-thought fine-tuning, reinforcement learning and use of synthetic data.
The innovation highlights how AI can build itself efficiently and drop costs by improving upon or using existing techniques. It will also set off a race by the leading AI labs to deliver AI performance via APIs, into the enterprise, at lower costs. Most critically, it opens up the aperture for value accrual at the application layer (to be covered in a future post).
2. The Case for Smaller, Specialized Models
Contrary to popular belief, smaller domain-specific models, tailored to specific domains or tasks, often outperform larger, general-purpose models for enterprise use cases, while requiring fewer resources to train and operate. Companies like Arcee.ai are pioneering this approach, offering platforms that enable organizations to build and maintain their own AI models on top of open-source general intelligence. This strategy allows enterprises to extend the capabilities of base models through parameter space adaptation, resulting in more efficient and cost-effective AI solutions. Others, such as MosaicML, acquired by Databricks in 2024 and utilized as part of the Databricks platform, have demonstrated that models trained on specific business domains can achieve superior results compared to larger models like GPT-3.5, while significantly reducing computational costs.
3. Bringing Data to Models: A Paradigm Shift
One of the most promising avenues for reducing AI deployment costs is the concept of bringing data to models, rather than the traditional approach of moving models to where the data resides. This paradigm shift can significantly reduce data transfer costs and latency while enhancing data privacy and security.
4. Optimized Inference Engines
Inference optimization directly impacts cost efficiency and latency in production. With AI training/inference costs outpacing revenue by 60-80%, a well-tuned inference engine that maximizes hardware utilization while minimizing compute resources is essential for sustainable AI deployment.
Together.ai has introduced an enterprise platform that claims to achieve 2-3x faster inference and up to 50% lower operational costs on existing cloud or on-premise infrastructure. Their continuous model optimization techniques, such as auto fine-tuning and adaptive speculators, help improve model performance over time.
5. GPU Orchestration and Resource Management
Effective GPU orchestration can reduce infrastructure costs by 40-50% through intelligent workload scheduling and resource allocation. Poor orchestration leads to GPU underutilization and bottlenecks, directly impacting your unit economics and ability to scale. Companies such as Fireworks.ai offer enhanced GPU orchestration capabilities, including job scheduling, auto-scaling, and traffic control. These features help enterprises maximize their GPU investments and optimize resource utilization.
6. Model Merging and Adaptation
Our portfolio company Sakana.ai, which is a frontier AI lab in Japan, has pioneered evolutionary model merge, an offering where the parameters of two language models are combined. This technique, along with continuous pretraining and alignment processes, allows organizations to create custom models that are both efficient and tailored to their specific needs.
B. The AI Data Transaction Layer
As enterprises grapple with the challenges of integrating AI into their existing systems and processes, I am seeing a new layer emerging to facilitate efficient and seamless data transactions between AI models and enterprise data sources. This AI data transaction layer is becoming increasingly critical for efficient and cost-effective AI deployments.
1. APIs for the AI-era
A new wave of AI-first API companies is revolutionizing how enterprises handle API development and software development kit (SDK) generation. These companies are creating sophisticated toolchains that significantly reduce development overhead, improve API consumption and time-to-market while improving API quality and maintainability. These platforms are transforming enterprise API development by:
- Automating repetitive development tasks
- Ensuring consistency across multiple programming languages
- Maintaining synchronization between APIs, documentation, and SDKs
- Providing enterprise-grade security and monitoring capabilities
Some interesting sub-segments include SDK generation and API management. For example, Stainless’ platform automatically generates high-quality SDKs in multiple languages including Python, TypeScript, Kotlin, and Go3. Others such as Speakeasy’s “Code as Docs” platform generate code samples directly from SDKs, streamlining technical documentation and implementation. Unkey provides specialized API management solutions with built-in rate limiting and token systems, crucial for AI service deployment.
2. Data Curation and Preparation
High-quality curated datasets are instrumental for model performance and competitive advantage. Without proper data preparation, proof-of-concepts won’t scale or demonstrate real value, regardless of model sophistication.
Our portfolio Scale.ai has positioned itself as a leader in this space, offering a comprehensive Data Engine that improves AI models by enhancing the quality and relevance of training data. Their suite of intelligent dataset management, testing, and model evaluation tools enables organizations to identify and label the most valuable data, maximizing the return on their labeling investments.
Similarly, companies such as Datology focus on automated data curation prioritizing high-impact training samples for generative AI models. Its techniques reduce redundant or low-quality data volumes, trimming training cycles by 20-30% and improving model accuracy by up to 30%.
3. Data Curation and Preparation
Complementary to curation and preparation, data compression could help with unit economics of deploying and running AI within the enterprise. Companies such as Granica, apply its ML-driven compression algorithms on data, reducing storage and transfer costs by up to 80% while accelerating query speeds by 56%. To clarify, data compression is a method for reducing the size of digital data by eliminating redundant or unnecessary information while preserving essential content. These algorithms enable efficient storage and faster transmission of data. By losslessly shrinking petabyte-scale AI training data in cloud object stores, enterprises lower their infrastructure expenses without sacrificing data integrity.
C. The Path Forward for Enterprise AI Adoption
As the AI landscape continues to rapidly evolve in 2025, there are several key opportunities to help enterprises maximize the value of their AI investments and move toward AI efficiency:
- Embrace Smaller, Specialized Models: Rather than defaulting to large, general-purpose models, organizations should explore the benefits of smaller, domain-specific models that can be more easily fine-tuned and deployed.
- Standardization and Interoperability: The industry will move toward standardized interfaces and protocols for AI infrastructure, making it easier for enterprises to switch between different providers, combine multiple specialized services and maintain consistent performance across platforms.
- Automated Infrastructure Optimization: Leveraging cloud-native AI platforms and optimized inference engines can significantly reduce operational costs and improve performance through features such as continuous model performance monitoring, automatic resource scaling and intelligent workload distribution.
- Invest in Data Quality: The adage “garbage in, garbage out” holds true for AI. Enterprises should prioritize data curation, cleansing, and preparation to ensure their AI models are trained on high-quality, relevant data.
- Adopt Flexible Deployment Strategies: Embracing hybrid deployment models that allow for both cloud and on-premises AI operations can help balance cost, performance, and data security considerations.
- Focus on the AI Data Transaction Layer: Developing a robust infrastructure for seamless data flow between enterprise systems and AI models is crucial for long-term success.
The next frontier in enterprise AI adoption lies in optimizing the efficiency of AI operations and streamlining the data transaction layer in order to get true ROI on these investments. Companies that focus on these aspects rather than just model selection will be better positioned to achieve sustainable AI adoption. The emergence of specialized and integrated platforms focusing on infrastructure optimization and data management suggests that the industry is moving toward more efficient, cost-effective solutions for enterprise AI deployment.
If you know a founder building in this space, we at Geodesic would love to hear from them.