Cybersecurity in an AI-First World
Written byWill Horyn
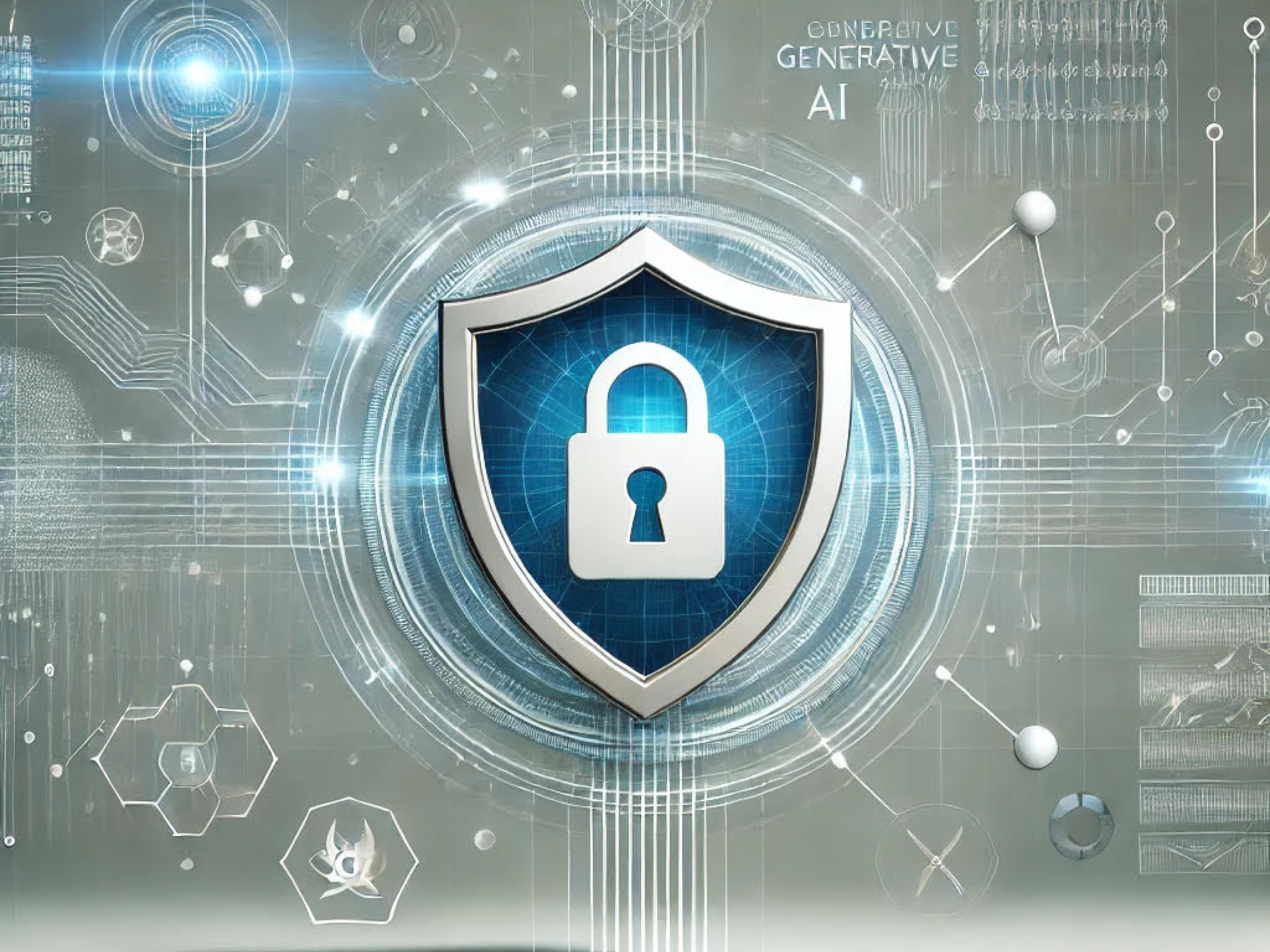
Generative AI (“GenAI”) is the buzziest term in enterprise technology right now and for good reason. With the potential to unlock new capabilities, leverage siloed proprietary data, and uplevel employees across the organization regardless of technical ability, the promise of this software is tangible. However as enterprises gradually shift from experimentation to production workloads, the excitement around GenAI is counterbalanced by an equally pressing consideration: cybersecurity risk. Every technological evolution introduces new vulnerabilities, risks, considerations — and solutions (we’ll touch upon that shortly). The shift to cloud demonstrated this aptly, catalyzing entirely new cybersecurity categories including Secure Access Service Edge (Netskope, Zscaler), Cloud Security Posture Management (Wiz, Orca, Palo Alto Networks), and Identity and Access Management (Okta, Transmit Security).
GenAI is no exception, and enterprises are actively voicing their concerns. HiddenLayer’s inaugural AI Threat Landscape Report indicates 89% of IT security leaders are concerned with security vulnerabilities associated with integrating third-party AI tools and models, while 75% believe these third-party AI integrations pose a greater risk than existing threats. In a recent Cisco poll, >50% of respondents indicated security concerns (specifically around data privacy and data loss prevention) as their top challenge to adopting AI/ML, ahead of operational aspects such as integration and day-to-day management. GenAI can also be used offensively by attackers, with Gartner estimating that by 2025 autonomous agents will drive advanced cyberattacks that give rise to “smart malware.”
Our own conversations with large enterprises validate the level of focus on this issue with commentary spanning a lack of internal safeguards as well as under-allocated budget:
- Director of Product Management at Public Cybersecurity Vendor: “The lack of maturity in the creation of AI systems [has surprised me the most]. Software has more established best practices. AI understanding and maturity is not yet in place, posing risks as it’s used/adopted. One vulnerability in the underlying model makes all downstream apps vulnerable.”
- CISO at Major Cruise Line Operator: “GenAI can be an accelerator for the company but can surrender IP if not handled correctly.”
- Sr. Principal Data Scientist at Large DC & Colocation Provider: “GenAI has caused a spur of requests for use cases. The barrier to spin-up LLMs has become low, so the need for security becomes high. There is a lot of attention [on GenAI security], but today it is a small portion of the budget; I expect budget allocation to grow going forward.”
GenAI-related risk manifests across two core forms: enhancement of existing attack vectors and enablement of new vulnerability exploitation. In terms of the former, familiar threats such as phishing, malware, and DDoS (Distributed Denial-of-Service) are augmented by AI, leading to more sophisticated attacks including robust social engineering and deep fakes, metamorphic malware, and empowered threat actors. Regarding the latter, a whole host of new challenges have materialized with the rise in GenAI adoption spanning data privacy, regulatory compliance, shadow AI, and LLM and application security. In particular, new tactics including data poisoning, prompt injection, and LLM DoS are being exploited to exfiltrate sensitive data, coerce manipulated outputs, and cause model outages or drive outsized inference costs (see OWASP’s Top 10 list for a broader set of LLM-related risks). Anthropic recently explored a form of prompt injection known as “jailbreaking” in this write-up, illustrating just one of the many ways in which LLMs and related applications are vulnerable.
Fortunately, there is reason for optimism. Scores of founders are answering the call and developing solutions to address these newfound challenges. Below we map out many of the startups operating across different layers of the GenAI cybersecurity stack today. Given how fast this space is evolving the landscape will surely look different even months from now, but this hopefully illustrates the level of activity and core areas of focus vendors are addressing in response to enterprise needs.
We have segmented the market across four key sub-sectors (with one bonus area) which we’ll dig into below. Note that some vendors span multiple sub-sectors given the range of capabilities available today. We suspect feature sets will continue to converge as vendors seek to offer an end-to-end platform approach where enterprises can manage all of their GenAI-related cybersecurity needs in one place.
- The Security layer is split across two core focus areas: Model Development and Model Runtime
-
- Model Development is the furthest upstream component of the GenAI cybersecurity stack and protects against risks before a model is put into production. This includes hardening the model against potential vulnerabilities and ensuring fidelity of underlying data with capabilities such as penetration testing, model scanning, vulnerability assessments, data validation and security (to protect against data poisoning), model supply chain security, and shadow AI discovery. Vendors such as Protect AI and HiddenLayer offer various features including robust model scanning and risk detection to secure pre-production environments. Ensuring model security upfront is critical as any vulnerability or impairment will cascade to all downstream applications.
- Model Runtime vendors such as Robust Intelligence and Lakera secure the model and related outputs when in production, whether for internal or external use cases. Critical functions include LLM firewalls (both on model input and output) and real-time detection & response. LLM firewalls are particularly effective in defending against prompt injection attacks, as they are able to filter or reject requests before they hit the model or block inappropriate model responses as a last resort. PS – if you want to try your hand as an LLM hacker, check out Lakera’s prompt injection challenge (suffice it to say I’ll be sticking to my day job).
-
- The Evaluation, Observability, and Monitoring space enables enterprises to ensure GenAI models perform as expected, remain reliable, and continuously improve over time. This is particularly important given the non-deterministic nature of GenAI where the same inputs can yield different outputs, and is further exacerbated by the difficulty in troubleshooting these models given their “black box” nature. Arize and Patronus AI are among the vendors in this space building platforms focused on model evaluation and observability at scale. Enterprises must have consistent insight into uptime and performance to mitigate model drift and hallucination risk as well as ensure high-fidelity outputs and compliance with standards and policies.
- Startups innovating in the Governance, Compliance, and Guardrails sub-sector are tackling a wide range of challenges with objectives broadly focused on ensuring ethical use, regulatory compliance, transparency, and policy adherence. This includes approaches to data privacy (e.g. homomorphic encryption, differential privacy, and synthetic data for PII redaction), data loss prevention, role-based access control (including RAG authentication/authorization), compliance with international and local standards, and establishing records for auditability. Credo and Nightfall are addressing this space by focusing on a centralized governance platform and data security, respectively.
- The core sub-sectors described above reflect the bulk of activity in this space thus far and largely equip cybersecurity practitioners to better protect GenAI models and applications against new threat vectors. There is however an exciting new area of innovation gaining traction: AI Agents. Built upon the premise of “fighting AI with AI,” agentic workflows can be used to execute increasingly sophisticated tasks and meaningfully reduce the burden on human cybersecurity practitioners who are already overwhelmed and in short supply. For example, Dropzone AI is pioneering the development of AI Security Operations Center (SOC) analysts that can autonomously triage the deluge of alerts human analysts are currently buried under. While early, this space is immensely promising and we expect to see many more vendors competing here in the near future.
Organizations of all sizes must bear in mind the increased threats stemming from GenAI. Those that choose to implement GenAI technologies themselves (whether proprietary or third party) should allocate appropriate budget to ensure their use is secure, compliant, accurate, and monitored. While this space will continue to evolve rapidly, we recommend enterprises reference the market map above and begin engaging with vendors to formulate their GenAI cybersecurity strategy.
At Geodesic we have been privileged to partner with cybersecurity pioneers such as Netskope, Snyk, Tanium, Duo, Transmit Security, Pindrop and Traceable, and are excited to work with the next wave of industry leaders. If you are building in this space, please do reach out – we’d love to learn more about your vision and compare notes on the future of the GenAI cybersecurity stack!